Understanding Big Data Analytics: The Future of Data Analysis

- What is Big Data Analytics?
- Why is Big Data Analytics important?
- The Benefits of Big Data Analytics:
- Big Data Analytics Techniques:
- 1. Data Mining:
- 2. Machine Learning:
- 3. Natural Language Processing (NLP):
- 4. Predictive Analytics:
- Big Data Analytics Tools:
- 1. Hadoop:
- 2. Spark:
- 3. Tableau:
- 4. SAS:
- Big Data Analytics Applications:
- 1. Healthcare:
- 2. Retail:
- 3. Finance:
- 4. Manufacturing:
- Big Data Analytics Lifecycle:
- 1. Data Acquisition:
- 2. Data Preparation:
- 3. Data Processing:
- 4. Data Analysis:
- 5. Results Presentation:
- Conclusion:
- Sources:
- Related Queries:
What is Big Data Analytics?
Big Data Analytics refers to the application of advanced data analytics tools, technologies, and techniques to analyze vast, complex, and diverse datasets to uncover valuable insights and gain a competitive advantage. The primary objective of Big Data Analytics is to extract actionable intelligence from data that would otherwise remain hidden when utilizing traditional data management tools.Why is Big Data Analytics important?
The importance of Big Data Analytics stems from the fact that organizations worldwide generate vast amounts of data every day, and traditional data management tools cannot handle the sheer volume, velocity, and complexity of this data. Big Data Analytics tools enable businesses to unlock valuable insights from their data and make data-driven decisions that enhance competitiveness and improve operational efficiency. Organizations that implement Big Data Analytics gain a competitive advantage by discovering trends, opportunities and, risks that set them apart from their competitors.The Benefits of Big Data Analytics:
The benefits of Big Data Analytics can be broken down into the following categories:
Big Data Analytics Techniques:
Big Data Analytics encompasses a broad category of technologies, techniques, and tools that can be used to analyze massive volumes of data. In this section, we will outline the most common Big Data Analytics techniques:1. Data Mining:
Data Mining refers to the process of extracting valuable information from large datasets, uncovering hidden patterns and trends, and discovering relationships between data elements. Data mining techniques involve machine learning, pattern recognition algorithms, and statistical analysis.2. Machine Learning:
Machine Learning is a type of Artificial Intelligence that enables systems to learn and improve from experience without being explicitly programmed. Machine learning techniques involve training algorithms to identify patterns and relationships within data, allowing the system to make predictions, automate decisions, and adapt to changing circumstances.3. Natural Language Processing (NLP):
Natural Language Processing is a subfield of Artificial Intelligence that deals with the interaction between humans and machines using natural language. NLP techniques involve processing and analyzing large volumes of text data to uncover trends, patterns, and relationships between data elements.4. Predictive Analytics:
Predictive Analytics is the process of using historical data to predict future outcomes and trends. Predictive Analytics techniques involve statistical modeling, machine learning, and data mining to identify patterns and relationships within data, allowing businesses to make predictions and take proactive measures to mitigate risks and improve operational efficiency.Big Data Analytics Tools:
To analyze vast amounts of complex data, organizations require advanced data analytics tools capable of processing and analyzing these massive datasets. The following are some of the most commonly used Big Data Analytics tools:1. Hadoop:
Hadoop is an open-source software framework that enables distributed storage and processing of large datasets across clusters of commodity hardware. Hadoop consists of two main components: Hadoop Distributed File System (HDFS) and MapReduce, a programming model used to process large amounts of data in parallel.2. Spark:
Apache Spark is an open-source data processing engine built for speed, ease of use, and advanced analytics. The Spark engine combines batch processing, real-time processing, and machine learning capabilities across diverse datasets, making it an ideal tool for Big Data Analytics.3. Tableau:
Tableau is a data visualization tool that enables businesses to create interactive dashboards and reports. Tableau connects to diverse data sources and allows users to analyze and visualize large datasets to uncover insights and make data-driven decisions.4. SAS:
SAS is a software suite that offers a broad range of data management, data analytics, and Business Intelligence tools. SAS enables users to access, manage, and analyze large datasets quickly and efficiently, providing insights that drive informed decisions.Big Data Analytics Applications:
Big Data Analytics has endless applications in various industries, some of which include:1. Healthcare:
Big Data Analytics systems are used in the healthcare industry to improve patient outcomes, reduce costs and enhance operational efficiency. For instance, Big Data Analytics tools can be used to monitor patient health, reduce readmission rates, detect fraud, and optimize resource allocation.2. Retail:
Big Data Analytics tools can be used to gain insights into customer behavior, preferences, and purchase patterns, thus enabling businesses to create targeted marketing campaigns, optimize pricing, and improve customer satisfaction.3. Finance:
Big Data Analytics systems are used in the Finance industry to detect fraud, mitigate risks, and optimize business operations. For instance, Big Data Analytics can be used to monitor financial transactions, detect unusual patterns, and improve operational efficiency.4. Manufacturing:
Big Data Analytics tools are used in the manufacturing industry to optimize production processes, reduce costs, and improve product quality. For instance, Big Data Analytics can be used to monitor machines, detect potential failures, and improve supply chain management.Big Data Analytics Lifecycle:
The Big Data Analytics Lifecycle is a framework that describes the stages involved in implementing a successful Big Data Analytics project. The lifecycle consists of the following stages:1. Data Acquisition:
The first stage of the lifecycle involves identifying the data sources and acquiring the datasets necessary for the analysis.2. Data Preparation:
The second stage involves cleaning, transforming, and organizing the data to convert it into structured data that can be analyzed.3. Data Processing:
The third stage involves processing and analyzing the data using advanced Big Data Analytics tools and techniques.4. Data Analysis:
The fourth stage involves analyzing the data to uncover valuable insights and make informed decisions.5. Results Presentation:
The final stage involves presenting the results of the analysis and making recommendations to stakeholders.Conclusion:
In conclusion, Big Data Analytics has revolutionized the way organizations make decisions, enabling them to extract actionable insights from vast amounts of data to gain a competitive advantage. Big Data Analytics offers a broad range of benefits, including improved decision-making, enhanced customer experience, better risk management, increased operational efficiency, and new revenue streams. With the right combination of Big Data Analytics tools, techniques, and expertise, businesses can embark on a successful Big Data Analytics project that yields valuable insights and drives informed decisions.Sources:
Related Queries:
Learn More :
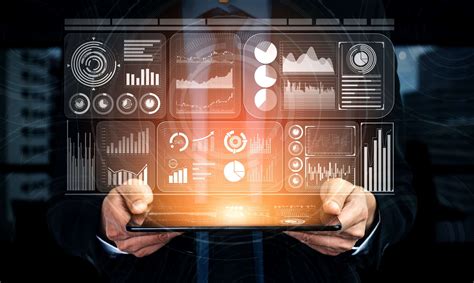
Every business generates a vast amount of data regularly. In recent years, the IT industry has seen a significant rise in the amount of data generation, and this data is growing at an unprecedented pa...
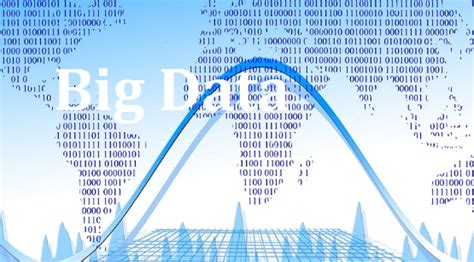
Big data is a buzzword that refers to the vast and complex datasets that traditional data management tools are unable to handle. With the rise of digital technologies and the internet, data has become...

With the rapid advancements in technology, industries have been significantly transformed. One of the most impactful changes is the emergence of big data, which has revolutionized the way businesses o...
![Data visualization: definition, examples, tools, advice [guide 2021]](/image/apeoplesmaporg/what-is-an-example-of-big-data.jpg)
Big data is a term used to describe the massive volume of structured and unstructured data that organizations generate on a daily basis. The sheer volume of data can be overwhelming and difficult to p...

Big data is a term that refers to large, complex, and unstructured data sets that are too difficult to process and analyze using traditional data processing tools and techniques. The characteristics o...

The demand for big data engineers is on the rise as more and more companies recognize the importance of data-driven decision making. According to Glassdoor, big data engineers are among the top 15 hig...
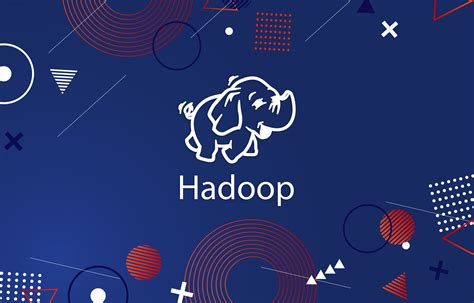
As technology keeps evolving, so does the amount of data generated on a daily basis. It's estimated that every single day, 2.5 quintillion bytes of data are created, and this number only keeps growin...
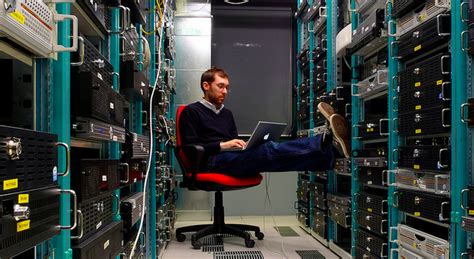
The following are some of the topics covered in a typical Big Data Engineering Syllabus:...

With the growing influence of data in today’s world, it’s important to understand what types of big data exist. Big data is defined as an expansive collection of data that is too complex to be pro...
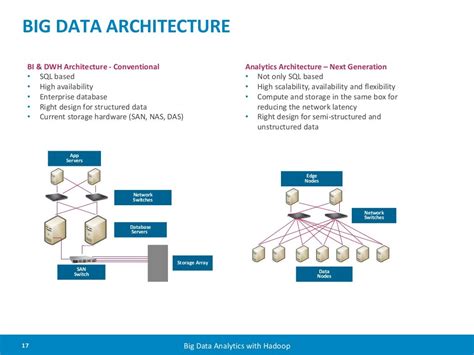
Big data is a term that refers to complex, large and disparate data sets that are too difficult to process using traditional data processing systems. These data sets not only involve structured but al...

In today's world, data is everything. Data has become a highly valuable asset for any organization that wants to succeed. How do organizations make sense of the enormous amount of data they generate o...
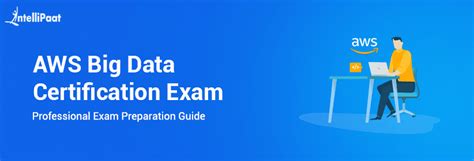
...
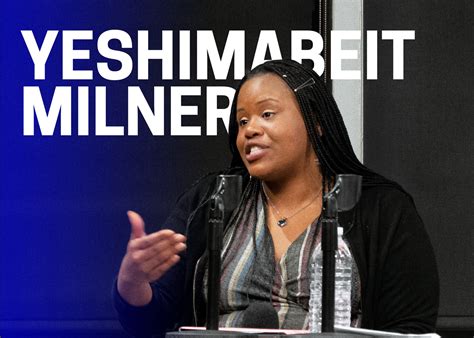
Big data has revolutionized the way we live our lives. From the way we shop to the way we communicate, big data has transformed the way we interact with the world around us. In the era of big data and...
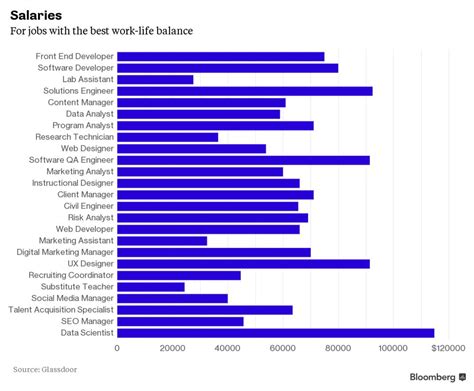
The world has taken a huge turn towards the digital age, where data is considered the new oil. With the increasing usage and importance of data analytics and big data, the demand for big data analysts...
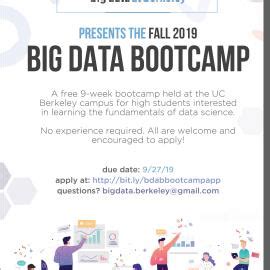
...
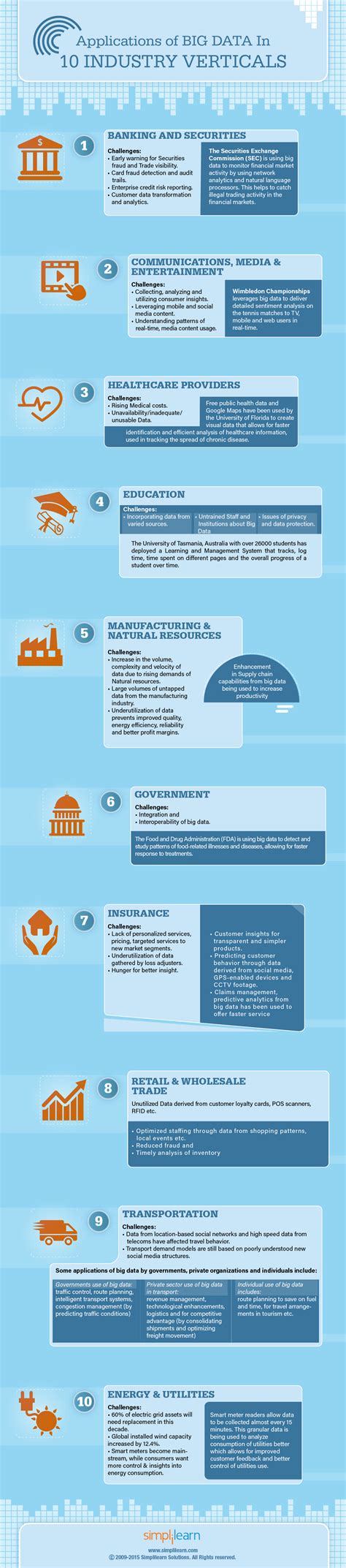
The amount of data being generated in the world today is growing at an unprecedented rate. From social media interactions to online purchases and electronic medical records, the variety, velocity, and...
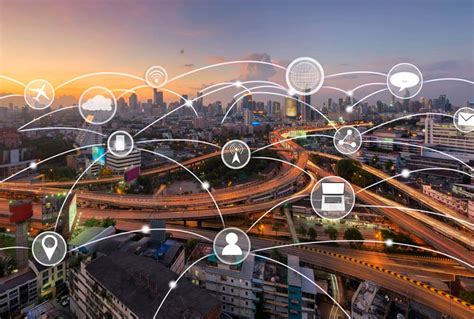
With the ever-increasing amount of data generated by businesses and organizations, the role of big data analysts has become more critical than ever before. Big data analysts are the professionals in c...
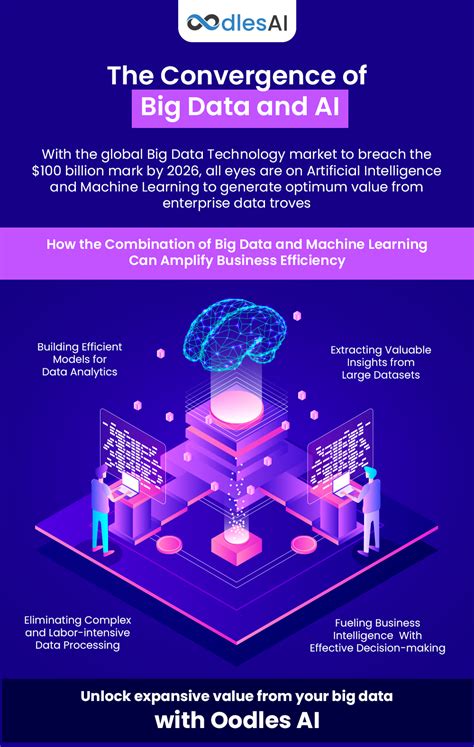
...
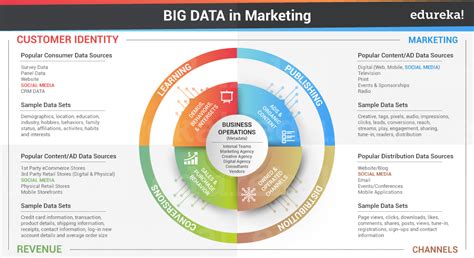
Big data has become one of the most talked-about technologies in recent years. The ability to process and analyze vast amounts of data has opened up a world of new possibilities and opportunities. Fro...
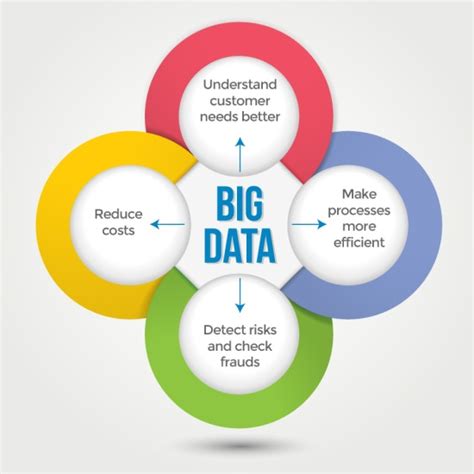
...