Hadoop Big Data: Understanding the Basics
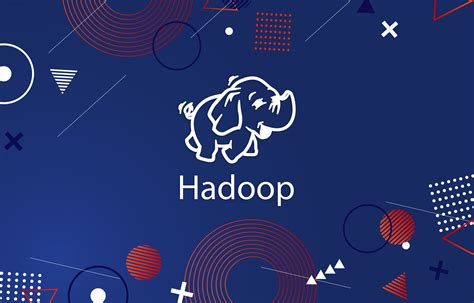
As technology keeps evolving, so does the amount of data generated on a daily basis. It's estimated that every single day, 2.5 quintillion bytes of data are created, and this number only keeps growing with each passing day. This data explosion means that traditional data processing tools and methods are no longer capable of handling the sheer amount of data being generated.
To process, store, and analyze this huge amount of data, a new set of tools and technologies have been developed, collectively referred to as Big Data. One of the most popular platforms used for Big Data analysis is Hadoop.
What is Hadoop Big Data?
Hadoop is an open-source software framework that allows for the distributed processing of large datasets across clusters of computers. It was created by Doug Cutting and Mike Cafarella and was named after the toy elephant of Doug's son. Hadoop is based on Google's MapReduce programming model and Google File System (GFS), which were developed to handle Google's massive amounts of data.
Hadoop Big Data provides a simple and flexible framework for distributed data processing with an emphasis on scalability and fault tolerance. It enables users to store and process vast amounts of data quickly and efficiently over a huge number of commodity hardware nodes.
Hadoop Big Data Architecture
Hadoop architecture consists of four core components, each with a specific function. These components are:
Hadoop Big Data Platform
Hadoop Big Data platform includes several modules that help manage and process data, ensuring smooth data analysis and extraction.
Hadoop Big Data Interview Questions
Here are some common interview questions related to Hadoop Big Data:
Hadoop Big Data Course
If you're interested in learning more about Hadoop Big Data, many online courses are available. Some popular ones include:
Hadoop Big Data Projects
Here are some project ideas to get started with Hadoop Big Data:
Hadoop Big Data Framework
Hadoop Big Data framework includes various tools and technologies to manage and process data. Here are some of the most popular ones:
Hadoop Big Data Tools
Here are some tools used for Hadoop Big Data processing:
Hadoop Big Data PDF
There are many resources available on Hadoop Big Data in PDF format. These include:
Conclusion
Hadoop Big Data allows for the efficient processing, storage, and analysis of large datasets using commodity hardware. It includes several components and modules that work together to create a distributed and fault-tolerant system. Hadoop Big Data is a growing field that offers many opportunities for developers, data scientists, and analysts.
Learn More :
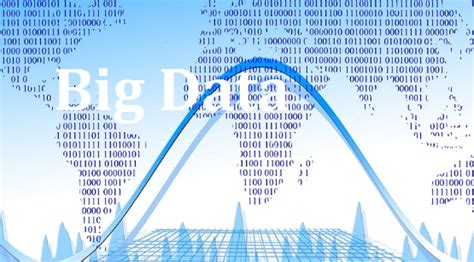
Big data is a buzzword that refers to the vast and complex datasets that traditional data management tools are unable to handle. With the rise of digital technologies and the internet, data has become...
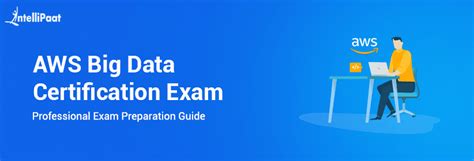
...
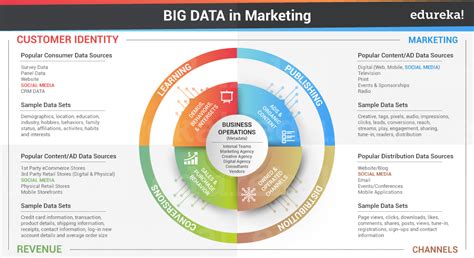
Big data has become one of the most talked-about technologies in recent years. The ability to process and analyze vast amounts of data has opened up a world of new possibilities and opportunities. Fro...
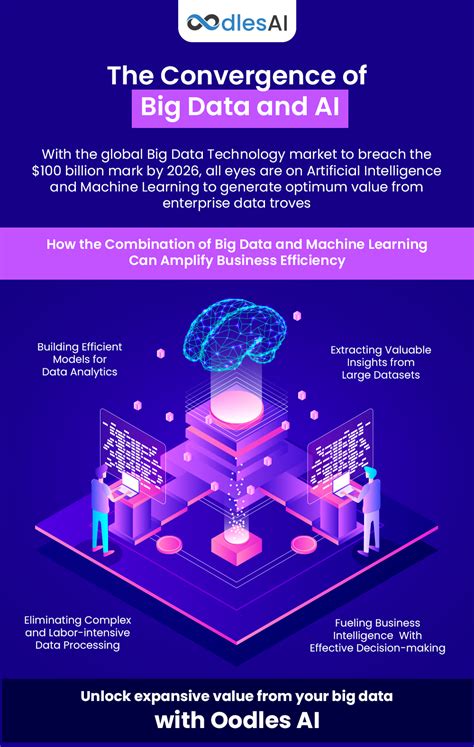
...
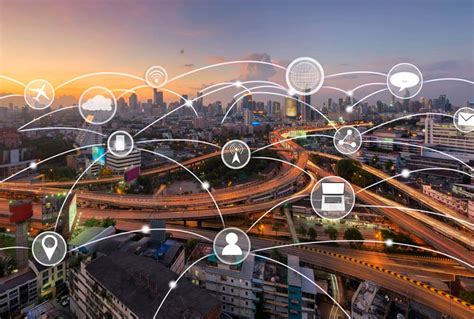
With the ever-increasing amount of data generated by businesses and organizations, the role of big data analysts has become more critical than ever before. Big data analysts are the professionals in c...
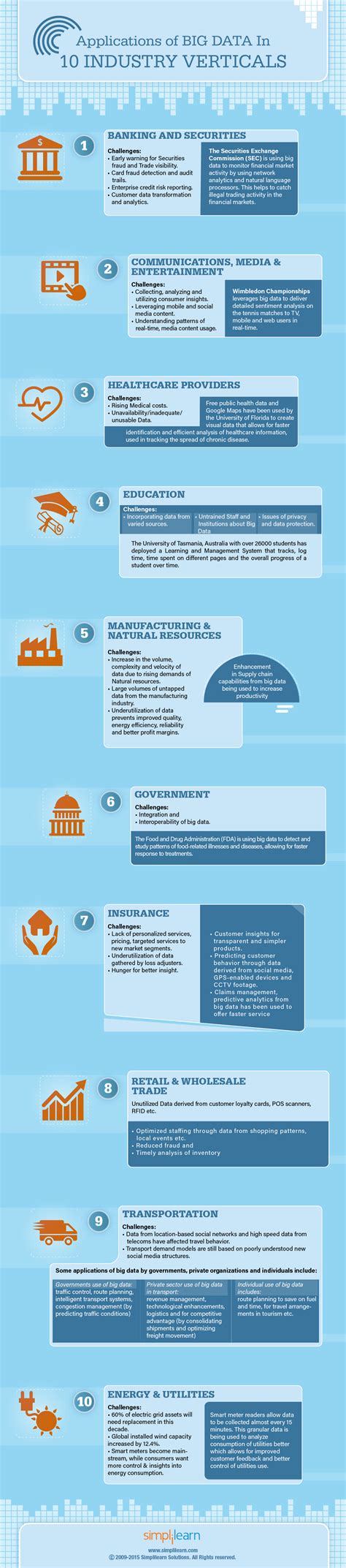
The amount of data being generated in the world today is growing at an unprecedented rate. From social media interactions to online purchases and electronic medical records, the variety, velocity, and...
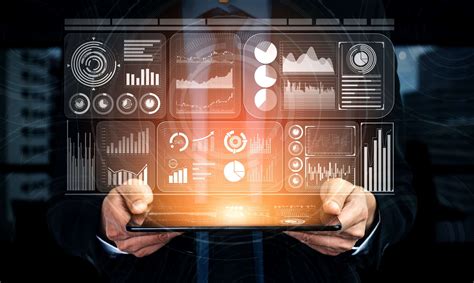
Every business generates a vast amount of data regularly. In recent years, the IT industry has seen a significant rise in the amount of data generation, and this data is growing at an unprecedented pa...
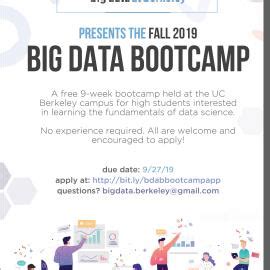
...
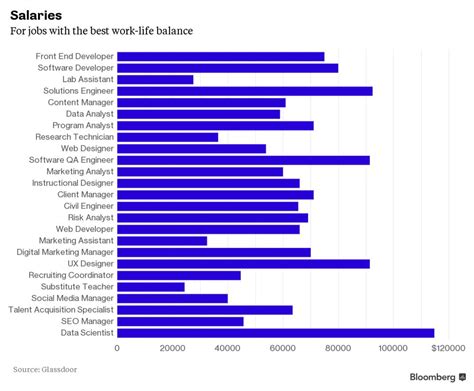
The world has taken a huge turn towards the digital age, where data is considered the new oil. With the increasing usage and importance of data analytics and big data, the demand for big data analysts...
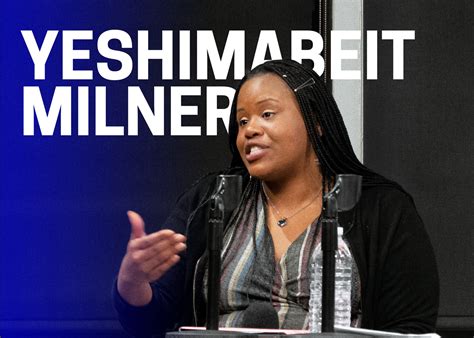
Big data has revolutionized the way we live our lives. From the way we shop to the way we communicate, big data has transformed the way we interact with the world around us. In the era of big data and...
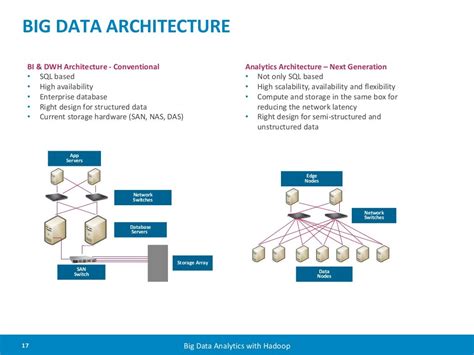
Big data is a term that refers to complex, large and disparate data sets that are too difficult to process using traditional data processing systems. These data sets not only involve structured but al...

In today's world, data is everything. Data has become a highly valuable asset for any organization that wants to succeed. How do organizations make sense of the enormous amount of data they generate o...

With the rapid advancements in technology, industries have been significantly transformed. One of the most impactful changes is the emergence of big data, which has revolutionized the way businesses o...

With the growing influence of data in today’s world, it’s important to understand what types of big data exist. Big data is defined as an expansive collection of data that is too complex to be pro...
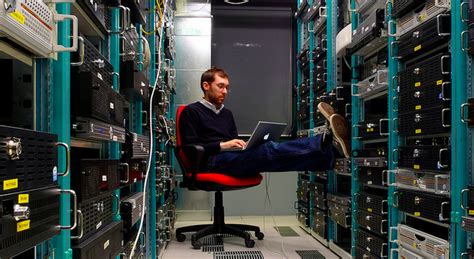
The following are some of the topics covered in a typical Big Data Engineering Syllabus:...

The demand for big data engineers is on the rise as more and more companies recognize the importance of data-driven decision making. According to Glassdoor, big data engineers are among the top 15 hig...

Big data is a term that refers to large, complex, and unstructured data sets that are too difficult to process and analyze using traditional data processing tools and techniques. The characteristics o...

The benefits of Big Data Analytics can be broken down into the following categories:...
![Data visualization: definition, examples, tools, advice [guide 2021]](/image/apeoplesmaporg/what-is-an-example-of-big-data.jpg)
Big data is a term used to describe the massive volume of structured and unstructured data that organizations generate on a daily basis. The sheer volume of data can be overwhelming and difficult to p...
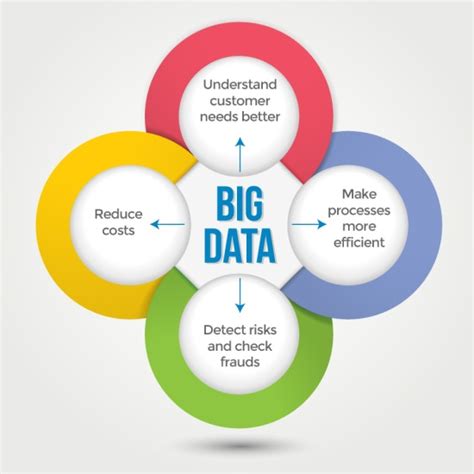
...